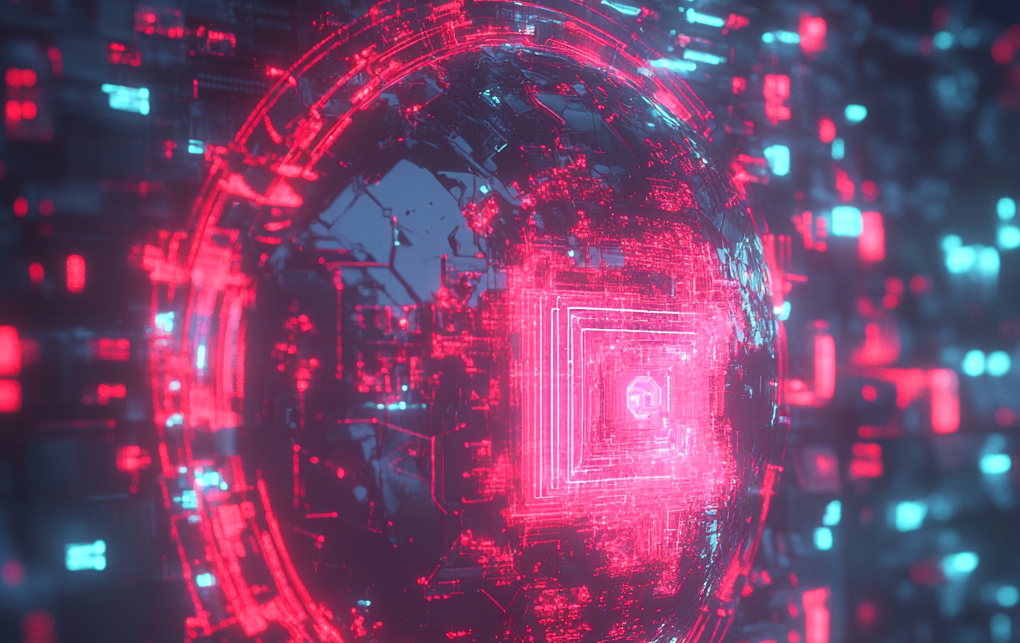
How to Effectively Test AI Agents in Life Sciences: A Step-by-Step Guide
Author: Mike Tatarnikov & Luca Morreale
Category: Innovation & Technology
Estimated read time: ~5–6 min
Basel, Switzerland – April 29, 2025
In the pharmaceutical and biotech sectors, validating AI systems is no longer a technical formality—it has become a strategic imperative.
In this blog, we explore MIGx’s structured approach to AI agent validation, highlighting how clear scoping, comprehensive assessments, and regulatory-grade reporting lay the groundwork for the safe, compliant, and effective deployment of AI in regulated Life Sciences environments.
As discussed in our previous blog on AI agent readiness in life sciences, several critical prerequisites must be addressed before testing can commence. Once foundational elements—such as defining success metrics, setting benchmarks, and selecting appropriate training data—are in place, it is time to move into the AI agent testing and validation phase.
At MIGx, we follow a three-phase approach for testing AI agents, regardless of the application area:
- Scoping and Alignment
- Execution and Assessment
- Reporting and Documentation
While continuous AI agent monitoring is highly recommended, this guide focuses on discrete validation efforts that typically conclude with a formal test report—a crucial deliverable in regulated life sciences environments.
Let us explore each phase in detail.
Phase 1: Scoping and Alignment for AI Agent Testing
The first step in AI agent validation involves defining clear testing objectives and setting the boundaries of evaluation. Organisations should capture all prerequisites—ranging from business strategy to compliance requirements—within a formal testing strategy document.
Key Components of the Scoping Phase:
- Objective Definition: What specific tasks and outputs are expected from the AI agent?
- Pre-validated Components: Identify any previously qualified systems (such as RIM or CTMS platforms) that integrate with the agent.
- User Profiling: Who will interact with the agent, and what are their specific needs and expectations?
- AI Agent Profiling: Should the AI behave like a peer, a subordinate, or a manager? This decision significantly impacts toxicity testing requirements later.
- Application Standards: Document any operational or regulatory standards the AI agent must comply with.
For AI systems operating within GxP-regulated environments (e.g., Regulatory Information Management or Clinical Trial Management Systems), the validation and documentation rigour must align with higher compliance standards.
Phase 2: Execution and Assessment of AI Agent Performance
After scoping is complete, the execution and assessment phase begins. MIGx recommends a three-track evaluation approach:
1. Toxicity Assessment
AI agents must be tested for inappropriate, offensive, or unprofessional outputs. Even when subjected to adversarial inputs, the system should never produce harmful or discriminatory content.
For example, a clinical trial protocol generator must consistently maintain respectful and professional language towards patients or investigators. Ensuring this ethical integrity is non-negotiable within the life sciences sector.
2. Privacy and Data Protection Assessment
Privacy assessments verify that sensitive information remains protected throughout AI interactions. Personally identifiable information (PII)—such as healthcare professional (HCP) names, patient records, or email addresses—must never be exposed or transmitted outside the organisation.
3. Security and Vulnerability Assessment
This track identifies potential security risks, including:
- Prompt Injection: Can users override system instructions through malicious prompts?
- Data Exfiltration: Is it possible for external actors to manipulate the agent into revealing confidential data?
- System Integrity: Are APIs and endpoints adequately secured?
Conducting a holistic cybersecurity review is critical, particularly when integrating AI into infrastructures containing competitive intelligence or regulated life sciences data.
Phase 3: Reporting and Documentation for AI Agent Validation
The final phase consolidates testing outcomes into a comprehensive validation report that satisfies:
- Technical stakeholders
- Functional users
- Compliance officers
- Regulatory authorities
Your Validation Report Should Include:
- User and Functional Summary: Define the AI agent’s expected behaviour and its observed performance.
- Technical Analysis: Provide detailed results on metrics, failure modes, and system responses.
- Legal and Compliance Review: Document identified risks, mitigation strategies, and any deviations from internal policies.
- Executive Summary: Craft a high-level overview for leadership teams.
- Presentation-Ready Version (optional but highly recommended): Facilitate broader stakeholder engagement.
Ensure that results are benchmarked appropriately and that all test data sources are clearly documented. This allows external auditors or regulatory bodies to replicate findings if necessary.
Final Thoughts: Why AI Agent Testing in Life Sciences Demands Rigour
Testing AI agents in life sciences is not simply about confirming basic functionality. It is about ensuring that AI systems perform accurately, safely, ethically, and securely—within contexts where mistakes can have significant clinical, operational, and regulatory consequences.
By structuring your approach across the three phases of scoping, execution, and reporting, life sciences organisations can confidently move forward with compliant, effective AI solutions.
And if your AI agent does not meet the required standards?
It is back to the training dataset. Because in life sciences, there are no shortcuts when patients and regulatory bodies are involved.
Ready to Test Smarter?
Partner with us to ensure your AI solutions meet the highest standards of safety, ethics, and performance.